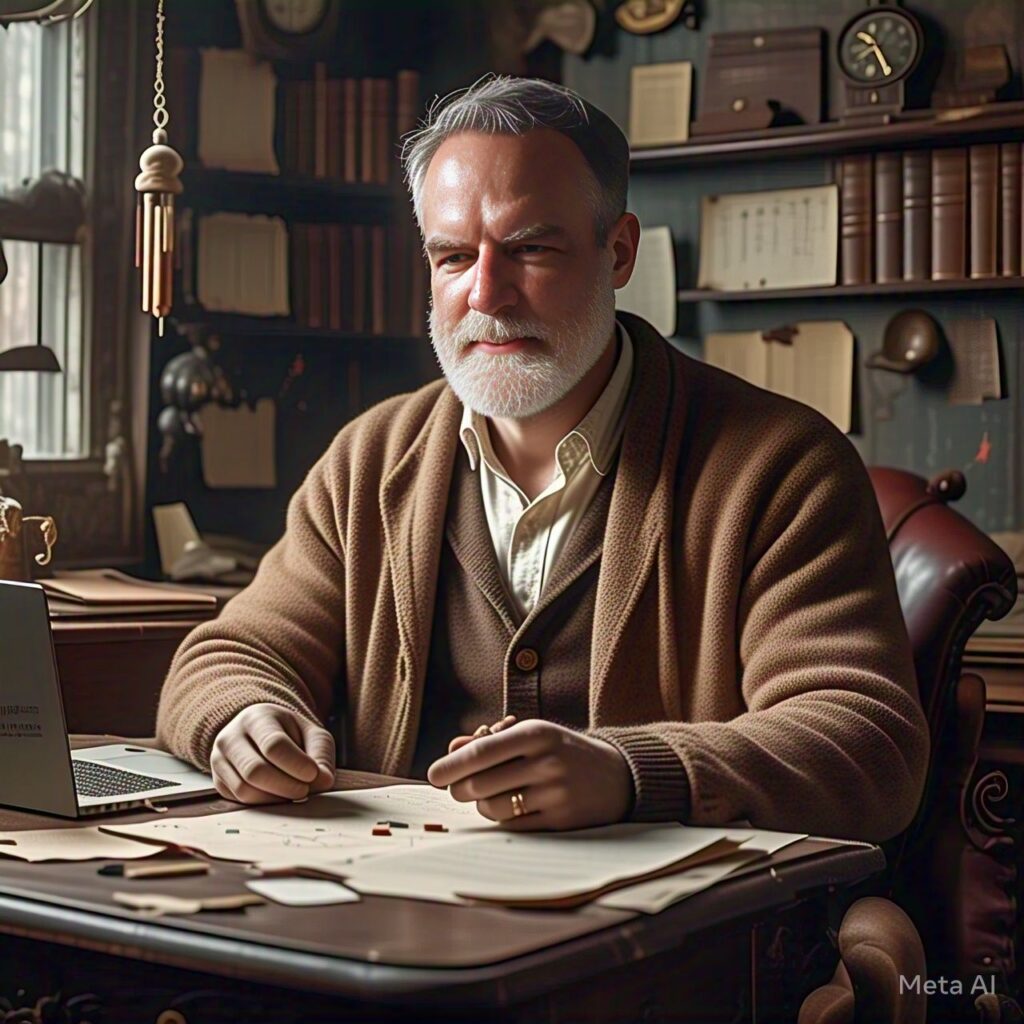
Will AI help me solve the complex problems I see around me?
AI can indeed be used to solve complex problems, and in some cases, even surpass human capabilities.
AI excels in pattern recognition, data analysis, and optimization, making it a powerful tool for tackling challenging scenarios.
: Spoiler – By allowing agents to collaborate, A2A unlocks the potential for creating more powerful and versatile agentic systems capable of solving complex problems.
One of the most recent groundbreaking trends in really complex problem-solving has been in migrating from MCP 0r Model Contex Protocol to A2A. A2A stands for Agent-to-Agent Interoperability Protocol.
Some of the groundbreaking improvements?
The two big ones are standardization, which allows interoperability between platforms, and Agents can now spawn new agents as needed.
Mr. Anderson, the agent spawn approaches.
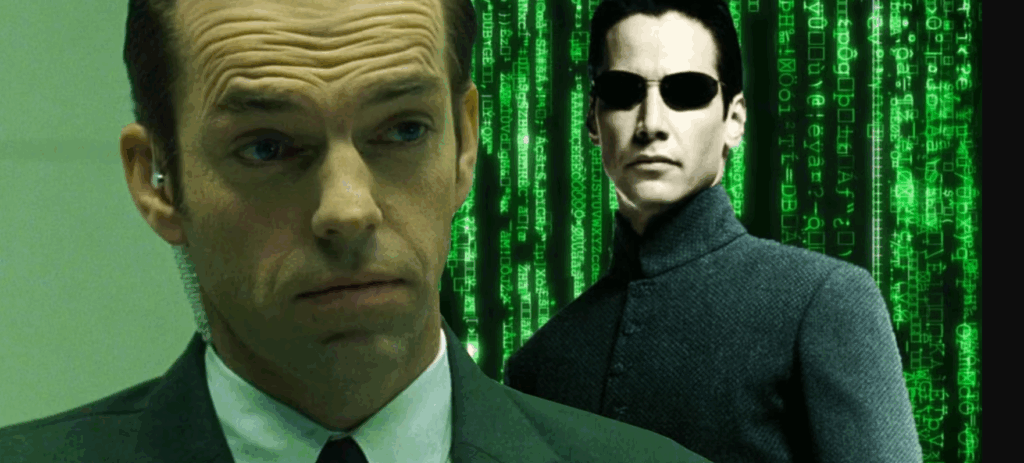
A standardized interoperability method that allows different AI agents, developed by various providers, to seamlessly communicate and collaborate. This interoperability is crucial for creating complex, multi-agent systems where agents can delegate tasks, share information, and work together to achieve a common goal.
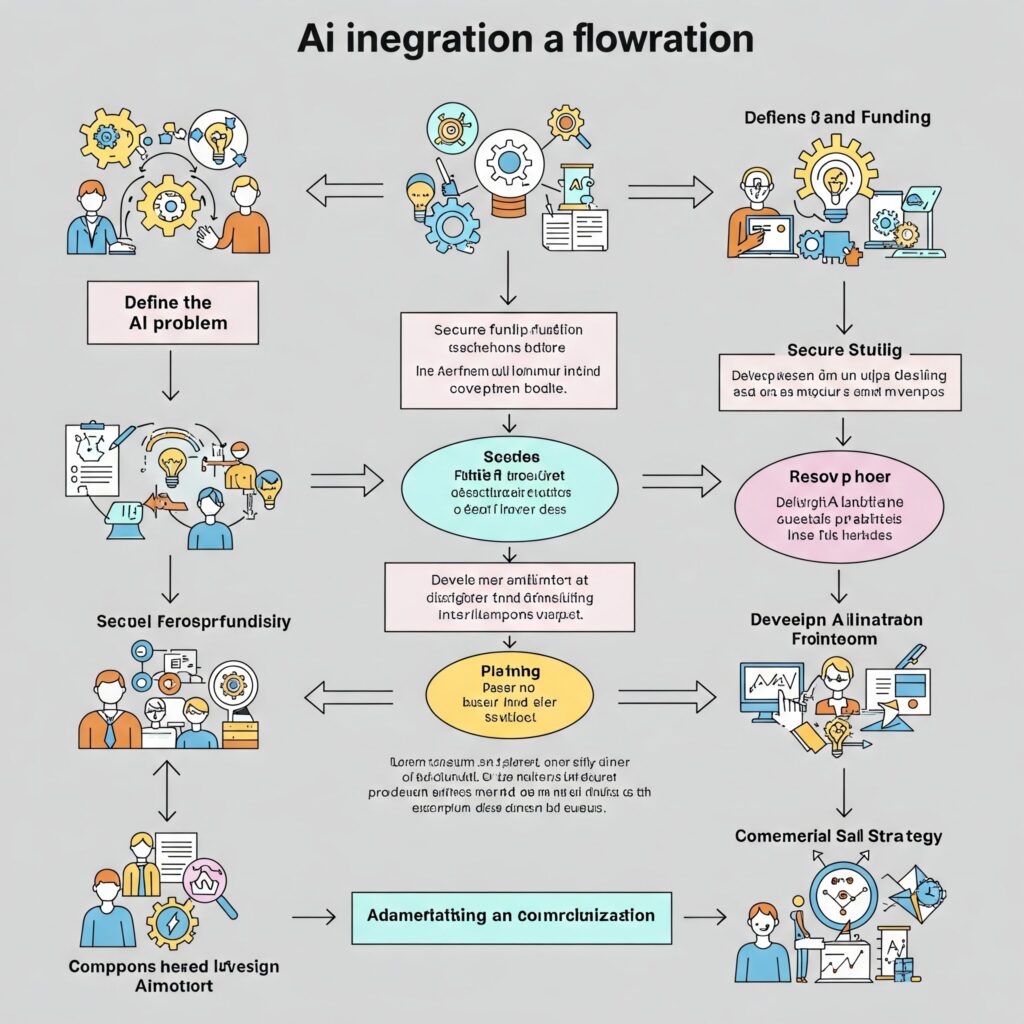
Importance of A2A in agentic workflows:
- Interoperability:
- A2A enables agents from different vendors to interact, regardless of their underlying technologies or architectures. This means developers can easily integrate agents from various sources into their workflows.
- Dynamic Collaboration:
- A2A fosters dynamic collaboration by allowing agents to self-organize, delegate tasks, and even spawn new agents as needed.
- Open-Ended Workflows:
- It doesn’t enforce rigid workflows, allowing for more flexible and adaptive solutions.
- Scalability:
- A2A facilitates the scaling of multi-agent systems, making it easier to manage a larger number of agents.
Importance of Standardization in agentic workflows:
- Standardization:
- A2A provides a standardized approach to managing and interacting with agents across different platforms and cloud environments.
- Enhanced Capabilities: By allowing agents to collaborate, A2A unlocks the potential for creating more powerful and versatile agentic systems capable of solving complex problems.
- Security:
- Enterprise-grade authentication and authorization.
In essence, A2A is a key enabler for the development of robust and flexible agentic workflows, allowing agents to work together seamlessly to achieve complex objectives. It’s a fundamental protocol for building the next generation of AI-powered applications.
Here’s how AI tackles complex problems:
- Algorithm Design and Optimization:
- AI algorithms can be designed to efficiently solve complex problems, such as optimizing logistics or finding the most efficient solutions in complex mathematical puzzles.
- Pattern Recognition and Identification:
- AI can analyze vast datasets to identify subtle patterns and anomalies that humans might miss, leading to breakthroughs in various fields like medicine, finance, and scientific research.
- Data Analysis and Processing:
- AI can handle large and complex datasets, automate data cleaning, and generate insights that would be difficult or time-consuming for humans to achieve.
- Predictive Modeling:
- AI can learn from historical data to forecast future trends and outcomes, enabling better decision-making in fields like finance, healthcare, and retail.
- Automated Problem Solving:
- AI systems can automate tasks, freeing up human resources to focus on more strategic or creative aspects of problem-solving.
- Collaboration and Augmentation:
- AI can work collaboratively with humans, providing insights, recommendations, and data-driven support to enhance problem-solving capabilities.
Examples of AI solving complex problems:
- Mathematical puzzles:
- AI systems like Fun Search have demonstrated the ability to find innovative solutions to complex mathematical problems, even surpassing human performance in some cases.
- Drug discovery:
- AI algorithms are being used to analyze vast amounts of data to identify potential drug candidates and accelerate the drug discovery process.
- Logistics and supply chain optimization:
- AI-powered systems are being used to optimize routes, manage inventory, and improve efficiency in complex logistics and supply chain operations.
- Predictive maintenance:
- AI can analyze data from machinery to predict potential failures and optimize maintenance schedules, reducing downtime and improving efficiency.
- Personalized education:
- AI can be used to personalize learning experiences and provide tailored support to students, improving learning outcomes and engagement.
Limitations:
While AI is a powerful tool, it’s important to acknowledge its limitations:
- Contextual Understanding:
- AI may struggle with situations requiring nuanced contextual understanding, common sense reasoning, or complex social interactions.
- Generalizability:
- AI models often perform best on specific tasks or datasets and may not generalize well to novel or unseen scenarios.
- Ethical Considerations:
- As AI becomes more prevalent, it’s crucial to address ethical considerations related to bias, fairness, and accountability.
Next Steps for Leaders with Very Complicated Problems?
1. Schedule an initial consultation with the DeReticular team
2. Identify a champion within your organization
3. Conduct a preliminary assessment of priority use cases
4. Explore available grant funding for AI technology
Contact Information For Leadership-specific inquiries:
Michael Noel
Email: Mike@BizBuilderMike.com
Phone: 480-442-3244
LinkedIn: linkedin.com/in/michaelnoel
Request a healthcare-specific demonstration of NVIDIA Clara capabilities to see the potential impact on your specific clinical workflows.
Leave a Reply
You must be logged in to post a comment.